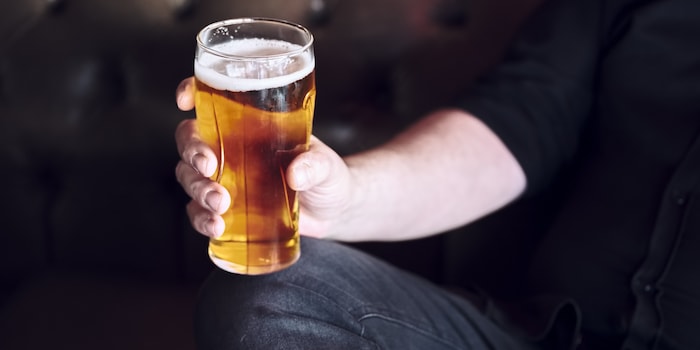
AI in medicine: could images of your knee reveal your love of beer?
Artificial intelligence (AI) purports to spot details in medical images that are hidden to the human eye. However, a new study has revealed how easy it is for AI models to identify misleading patterns in data and come to false conclusions.
Imagine if a computer program could use an X-ray of your knee to predict whether you enjoy drinking beer. Sound absurd? Well, this is exactly what researchers achieved during a recent study. But there was no celebration of this apparent success. Instead, the scientists who published the paper set out to draw attention to a serious problem in the development of AI in medical imaging.
The risk of shortcutting
The paper, published in Scientific Reports, demonstrates how susceptible deep learning algorithms are to so-called shortcutting. In other words, they draw upon superficial patterns in the training data instead of learning medical characteristics that’d actually be relevant.
Shortcutting occurs when an AI model finds a simple way to accomplish a task without truly understanding the underlying problem. In medicine, this can be especially problematic.
How AI connects knee X-rays to beer and bean preferences
To illustrate this problem, researchers trained simple neural networks to predict whether patients refrained from drinking beer or eating fried beans on the basis of knee X-ray images. With no logical way to identify these preferences from a knee X-ray, the AI shouldn’t have been able to make any predictions. The actual results, however, might surprise you. The AI models were able to predict patients’ preferences for beans down to an accuracy of 63 per cent and even 73 per cent for beer. That’s significantly more accurate than if they’d simply guessed. Plus, the AI came up with these results despite the fact that there’s no plausible medical connection between bean-eating or beer-drinking and knee appearance.
Hidden correlations in the data
So, how did the models arrive at these results? The answer lies in hidden correlations within the datasets. The AI systems learned to spot subtle patterns related to demographic factors or technical details of the X-ray image. In other words, instead of basing their predictions on the appearance of the X-ray images, they used the patients’ age, gender, ethnicity and place of residence. For instance, the AI managed to identify the clinical centre where the images were taken or even determine when the X-ray machine was manufactured.
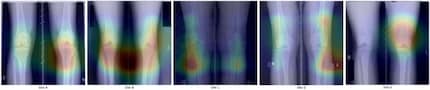
Source: Hill et al./Scientific Reports, CC-by-nc-nd 4.0
Why this is dangerous
The study authors warned that, «Shortcutting makes it trivial to create models with surprisingly accurate predictions that lack all face validity.» In medical research, this could lead to false conclusions. When you think an AI model has made a groundbreaking new discovery, it may have actually just found a random correlation within the data.
The problem of shortcutting goes far beyond simple distortions. This study shows that AI models not only use individual confounding factors such as gender or age, but also complex combinations of different variables. Even if you exclude obvious influencing factors, the algorithms often find other ways to make their predictions.
94 people like this article
Science editor and biologist. I love animals and am fascinated by plants, their abilities and everything you can do with them. That's why my favourite place is always outside - somewhere in nature, preferably in my wild garden.